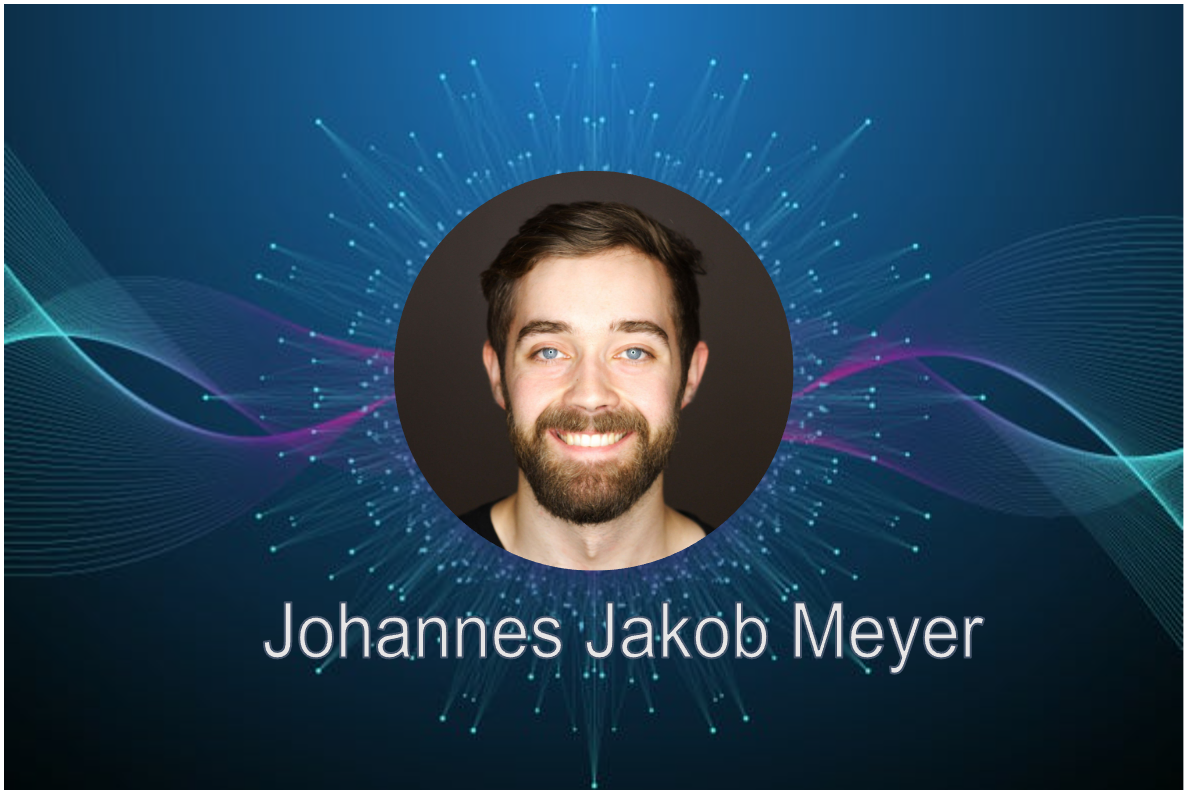
Mar
3
4:00pm
Exploiting Symmetry in Variational Quantum Machine Learning
By Quantum Formalism
Abstract
Variational quantum machine learning is an extensively studied application of near-term quantum computers. The success of variational quantum learning models crucially depends on finding a suitable parametrization of the model that encodes an inductive bias relevant to the learning task. However, precious little is known about guiding principles for the construction of suitable parametrizations. In this talk, I explore when and how symmetries of the learning problem can be exploited to construct quantum learning models with outcomes invariant under the symmetry of the learning task. I show how only very restricted classes of classical-to-quantum embeddings allow for a faithful representation of the symmetry that acts on the level of the data, a property which we call “equivariance”.
Speaker Bio
Johannes is a PhD student with Jens Eisert at the Dahlem Center for Complex Quantum Systems, Freie Universität Berlin. His research interests revolve around all facets of Quantum Information Theory, especially Quantum Computing on NISQ devices, Quantum Machine Learning and Quantum Metrology. Johannes also enjoys working on fundamental aspects like learning theory as well as working on more applied issues at the quantum-classical boundary.
hosted by

Quantum Formalism
share